Standard Guidance, Navigation, and Control (GN&C) systems take state data from a navigation system and create a trajectory that minimizes some a-priori determined cost function. These cost functions are typically time, money, weight, or any general physically realizable quantity. Previous work has been done to show the effectiveness of using risk as the sole objective function. However, this previous work used Poisson distributions and historical estimates to achieve this goal. In this paper we present the situation-risk assessment (SRA) method contained within the intelligent situation assessment and collision avoidance (iSC) platform. The SRA method uses data clustering, and pattern recognition to create a historically based estimate of guidance probabilities. These are then used in data driven, dynamic models to create the future probability fields of the situation. This probability, along with the other agent’s goals and objectives, are then used to create a minimum risk guidance solution in the nautical environment.
DYNAMIC PROBABILITY FIELDS FOR RISK ASSESSMENT AND GUIDANCE SOLUTIONS
1The University of Southern California
2The University of Southern California
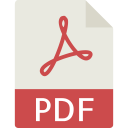
DOI: 10.1515/aon-2019-0004
ABSTRACT
KEYWORDS
REFERENCES
[1] Barwise J., “Scenes and Other Situations,” The Journal of Philosophy, vol 78, pp. 369-397, Oct. 2006.
[2] Devlin K., “Situation Theory and Situation Semantics”, Handbook of the History of Logic, vol 7, pp. 601-664, 2006.
[3] Endsley M., “Toward a theory of situation awareness in dynamic systems,” Human Factors: The Journal of the Human Factors and Ergonomics Society, vol 37, pp. 32-64, March 1995.
[4] Endsley M., “Design and Evaluation For situation Awareness Enhancement,” Proceedings of the Human Factors Society, pp. 97-101, 1998.
[5] Endsley M., “From Here to Autonomy: Lessons Learned From Human-Automation Research,” Human Factors: The Journal of Human Factors and Ergonomics Socieity, vol 59, pp. 5-27, Feb 2017.
[6] Fossen T., Marine Control Systems: Guidance, Navigation and Control of Ships, Rigs and Underwater Vehicles, Trondheim, Norway: Marine Cybernetics 2002.
[7] Kaiser M., Gans N., and Dixon W., “Vision-based estimation for guidance, navigation, and control of an aerial vehicle,” IEEE Transactions on Aerospace and Electronic Systems, vol 46, pp. 1064-1077, August 2010.
[8] Kokar M., Matheus C., and Baclawski K., “Ontology-Based Situation Awareness”, Information Fusion, vol 10, pp. 83-98, Jan 2009.
[9] Kutsuna K., Kuwahara S., Ando H., Nakamura S., and Nakashima T., “Feasibility Study of Automated Process of Ship Navigation A Framework of Action Planning System (APS)”, 2018 ICMASS, Korea, 2018.
[10] Lavertsky E., and Wise K. A., Robust and Adaptive Control: with Aerospace Applications, London: Springer-Verlag, 2013.
[11] McHenry R., Long A., Cockrell B., Thibodeau J., and Brand T., “Space Shuttle ascent guidance, navigation, and control,” J. of the Astronautical Sciences, vol. 27, pp. 1-38, January 1979.
[12] Ross I. M., and Karpenko M., “Review of Pseudospectral Optimal Control: From Theory to Flight”, Annual Reviews in Control, vol 36, pp. 182-197, 2012.
[13] Steinfeldt B., Grant M., Matz D., Braun R., and Barton G., “Guidance, Navigation, and Control System Performance Trades for Mars Pinpoint Landing,” J. of Spacecraft and Rockets, vol 47, pp. 188-198, January 2010.
[14] Ullman D., The Mechanical Design Process, New York, NY: McGraw Hill, 2010.
[15] Vanderplaats G., Numerical Optimization Techniques for Engineering Design, New York, NY: McGraw Hill, 1984.
[16] Williams E., and Jin Y., “Use of Situation and Risk Modeling in Guidance Solutions”, IEEE/ION Position Location and Navigation Symposium, 23-26 Apr. 2018.
[17] Williams E., and Jin Y., “Low Earth Orbit Debris Avoidance Using Situation-Risk Assessment Modeling”, 2018 AIAA SPACE Forum, 2018.
[18] Williams E., and Jin Y., “Development of Probability Fields for Collision Avoidance,” currently unpublished.